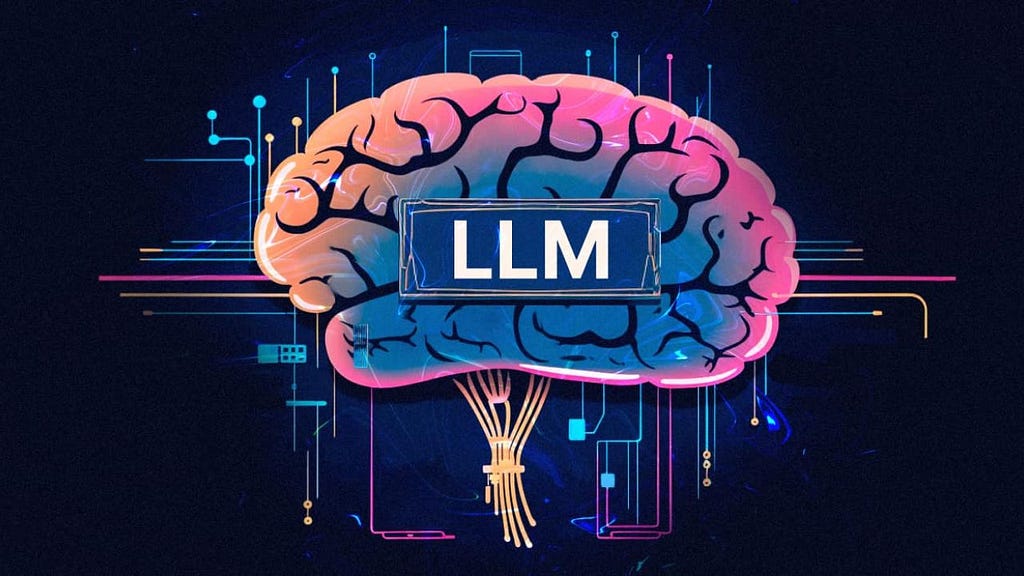
Fig: Large Language Models (LLM) Development
In the rapidly evolving landscape of artificial intelligence, Large Language Models (LLMs) have emerged as a transformative force. These models, built on sophisticated neural network architectures, are capable of understanding and generating human-like text, revolutionizing industries from customer service to creative writing. As we delve deeper into the age of digital transformation, the development of LLMs is becoming increasingly pivotal, offering unprecedented capabilities in natural language processing (NLP) and machine learning.
At the core of LLM development lies the ability to process vast amounts of textual data, enabling machines to comprehend context, infer meaning, and generate coherent narratives. This technological advancement is not just a leap in AI; it’s a paradigm shift that is redefining the way we interact with machines and, by extension, each other. From the initial stages of data collection and model training to the complexities of fine-tuning and deployment, the journey of LLM development is as intricate as it is groundbreaking.
In this introduction, we will explore the fundamentals of LLM development, the key challenges involved, and the potential these models hold for the future of AI-driven applications. Whether you are a developer, a business leader, or simply an enthusiast, understanding the intricacies of LLMs is crucial to navigating the next wave of AI innovation.
Overview of LLM and how it revolutionizes businesses?
Large Language Models (LLMs) are advanced artificial intelligence systems designed to understand, generate, and manipulate human language in a highly sophisticated manner. These models, such as OpenAI’s GPT-4 and Google’s BERT, are built using deep learning techniques, specifically leveraging vast neural networks with billions of parameters. LLMs are trained on extensive datasets, encompassing a wide array of text from books, articles, websites, and more, allowing them to grasp the nuances of language, context, and even idiomatic expressions.
The core strength of LLMs lies in their ability to generate text that is contextually relevant, coherent, and often indistinguishable from that written by humans. They can perform tasks such as language translation, summarization, content creation, and even code generation. As they continue to evolve, LLMs are becoming integral to various applications across industries, fundamentally altering how businesses operate and interact with technology.
How LLMs Revolutionize Businesses:Enhanced Customer Interaction:
- LLMs are transforming customer service by powering chatbots and virtual assistants that provide instant, accurate, and context-aware responses. This automation not only improves customer satisfaction by delivering quick support but also reduces the workload on human agents, allowing businesses to operate more efficiently.
Personalized Marketing and Content Creation:
- Businesses are leveraging LLMs to create personalized marketing campaigns and content at scale. These models can generate tailored product descriptions, emails, and advertisements that resonate with individual customer preferences, driving higher engagement and conversion rates.
Data Analysis and Decision Support:
- LLMs are adept at processing and analyzing vast amounts of textual data, enabling businesses to extract actionable insights from customer reviews, social media, and market trends. This capability supports more informed decision-making, helping companies stay ahead in competitive markets.
Streamlined Operations:
- By automating routine tasks such as document drafting, report generation, and even legal contract analysis, LLMs help businesses streamline their operations. This reduces human error, accelerates workflows, and allows employees to focus on higher-value tasks that require creativity and strategic thinking.
Innovation in Product Development:
- LLMs are also driving innovation by assisting in product development processes. From generating ideas for new products to refining existing ones through data-driven insights, these models empower businesses to innovate faster and more effectively.
Enhanced Collaboration:
- LLMs facilitate better collaboration across global teams by providing real-time language translation and communication tools. This capability breaks down language barriers, enabling seamless interaction and collaboration in multinational companies.
The integration of Large Language Models into business processes is revolutionizing how companies operate, communicate, and innovate. By harnessing the power of LLMs, businesses can enhance efficiency, deliver more personalized experiences, and make more informed decisions, ultimately gaining a competitive edge in the rapidly evolving digital economy. As these models continue to advance, their impact on the business world is set to grow even more profound, opening up new possibilities for innovation and growth.
LLM Development Services
As the capabilities of Large Language Models (LLMs) continue to expand, more businesses are recognizing the transformative potential of integrating these advanced AI technologies into their operations. LLM development services offer companies the expertise and tools needed to harness the full power of these models, enabling them to revolutionize their customer interactions, content creation, data analysis, and much more. Whether you’re looking to develop custom applications, optimize existing processes, or innovate new products, LLM development services can provide the foundation for success in the AI-driven marketplace.
Key LLM Development Services:
Custom LLM Model Development:
- Tailored to meet specific business needs, custom LLM development involves creating language models that are fine-tuned on industry-specific data. This ensures that the AI model is not only accurate but also contextually relevant, providing insights and outputs that align with the unique requirements of the business.
LLM Integration and Deployment:
- Seamless integration of LLMs into existing systems and workflows is crucial for maximizing their utility. LLM development services often include the deployment of these models within a company’s infrastructure, ensuring they operate efficiently and effectively within the business environment.
Fine-Tuning and Optimization:
- Fine-tuning existing LLMs on specific datasets allows businesses to enhance the model’s performance for particular tasks. LLM development services provide expertise in optimizing models to improve accuracy, reduce computational costs, and tailor outputs to the business’s precise needs.
Natural Language Processing (NLP) Solutions:
- Leveraging LLMs for various NLP tasks, such as text classification, sentiment analysis, and entity recognition, can provide businesses with powerful tools for analyzing and understanding large volumes of unstructured data. These services enable businesses to extract valuable insights from customer feedback, social media, and other text sources.
AI-Powered Content Creation:
- LLMs can be used to generate high-quality content, from marketing copy to technical documentation. Development services can create custom models that produce content aligned with brand voice and guidelines, enabling businesses to scale content production while maintaining consistency and quality.
Chatbot and Virtual Assistant Development:
- Advanced chatbots and virtual assistants powered by LLMs can provide personalized customer support, automate routine inquiries, and improve user engagement. Development services include designing, training, and deploying these AI-driven agents to meet the specific needs of a business.
Model Maintenance and Support:
- Continuous monitoring, updating, and maintenance of LLMs are essential for ensuring they remain effective as business needs evolve and new data becomes available. LLM development services typically offer ongoing support to keep models up-to-date and perform optimally.
Security and Compliance Consulting:
- Implementing LLMs within a business environment requires adherence to security standards and regulatory compliance. Development services include consulting on best practices for data privacy, security, and compliance, ensuring that AI models are deployed responsibly and ethically.
Benefits of Leveraging LLM Development Services:
- Enhanced Efficiency: Automating complex language-related tasks reduces manual effort, allowing employees to focus on higher-value activities.
- Scalability: Custom LLMs enable businesses to scale their operations, from customer service to content creation, without compromising quality.
- Innovation: By integrating cutting-edge AI, companies can drive innovation in product development, marketing, and customer engagement.
- Competitive Advantage: Businesses that leverage LLMs can gain a significant edge over competitors by offering more personalized, efficient, and insightful services.
LLM development services provide businesses with the tools and expertise necessary to fully capitalize on the capabilities of Large Language Models. From custom model creation to integration, fine-tuning, and ongoing support, these services empower companies to unlock the potential of AI, driving growth and innovation in an increasingly digital world. Whether you are looking to enhance existing operations or pioneer new AI-driven solutions, LLM development services are the key to staying ahead in the competitive landscape.
Expertise in various AI Models
Artificial Intelligence (AI) has evolved into a powerful tool that drives innovation across industries. At the heart of AI’s success are various AI models, each designed to solve specific problems and cater to different applications. Having expertise in a wide range of AI models allows businesses and developers to choose the right tool for their unique needs, maximizing the potential of AI technologies. This guide explores the different types of AI models and the expertise required to leverage them effectively.
1. Large Language Models (LLMs):
- Overview:
- LLMs, such as GPT-4, BERT, and T5, are designed for natural language processing (NLP) tasks. These models can generate, understand, and analyze human language, making them ideal for applications like chatbots, content creation, and sentiment analysis.
- Expertise Required:
- Natural Language Processing: Understanding of NLP techniques, including tokenization, text embedding, and language modeling.
- Model Fine-Tuning: Ability to fine-tune pre-trained models on specific datasets to improve performance for particular tasks.
- Deployment: Skills in deploying LLMs within business applications, ensuring scalability and efficiency.
- Ethical Considerations: Knowledge of responsible AI practices to mitigate biases and ensure compliance with data privacy regulations.
2. Convolutional Neural Networks (CNNs):
- Overview:
- CNNs are specialized neural networks primarily used for image and video recognition. They are the backbone of computer vision applications, enabling tasks like object detection, facial recognition, and image classification.
- Expertise Required:
- Image Processing: Proficiency in preprocessing techniques like resizing, normalization, and augmentation.
- CNN Architecture: Understanding of layers such as convolutional, pooling, and fully connected layers, and how to design and optimize them.
- Transfer Learning: Ability to apply pre-trained models like ResNet, VGG, and Inception to new datasets for efficient training.
- Application Development: Skills in integrating CNNs into applications like security systems, medical imaging, and autonomous vehicles.
3. Recurrent Neural Networks (RNNs) and Long Short-Term Memory Networks (LSTMs):
- Overview:
- RNNs and LSTMs are designed to handle sequential data, making them ideal for time series prediction, speech recognition, and natural language processing tasks like translation and summarization.
- Expertise Required:
- Sequence Modeling: Understanding of how RNNs and LSTMs handle dependencies across time steps in sequential data.
- Training Techniques: Knowledge of techniques to prevent issues like vanishing gradients, which can affect long-term dependencies.
- Application in NLP: Skills in applying RNNs and LSTMs to tasks such as language modeling, speech-to-text conversion, and machine translation.
4. Generative Adversarial Networks (GANs):
- Overview:
- GANs are a class of AI models used to generate new data samples that resemble a given dataset. They are widely used in creative fields, such as generating realistic images, art, and even synthetic data for training purposes.
- Expertise Required:
- Model Architecture: Understanding of the generator and discriminator networks that form the core of GANs.
- Training Dynamics: Knowledge of how to balance the training of the generator and discriminator to achieve realistic outputs.
- Applications: Expertise in applying GANs to various domains, including image synthesis, style transfer, and data augmentation.
5. Transformer Models:
- Overview:
- Transformer models, such as the original Transformer, BERT, and GPT series, have revolutionized NLP by enabling parallel processing of data sequences. They are used in tasks like translation, summarization, and question-answering.
- Expertise Required:
- Attention Mechanisms: Deep understanding of self-attention and multi-head attention mechanisms that allow transformers to process sequences in parallel.
- Model Training: Ability to train large transformer models, often requiring specialized hardware and distributed training techniques.
- Fine-Tuning: Skills in fine-tuning transformers for specific NLP tasks, leveraging transfer learning for improved performance.
6. Reinforcement Learning Models:
- Overview:
- Reinforcement learning (RL) models are used in environments where an agent learns to make decisions by interacting with the environment. Applications include robotics, gaming, and autonomous systems.
- Expertise Required:
- Reward Systems: Understanding of how to design reward functions that guide the agent’s learning process.
- Exploration vs. Exploitation: Knowledge of strategies for balancing exploration of new actions and exploitation of known actions.
- Simulation and Training: Expertise in setting up environments for training RL models, including simulations and real-world scenarios.
7. Hybrid Models:
- Overview:
- Hybrid models combine different types of AI models to leverage their strengths. For example, combining CNNs with RNNs for video analysis or integrating LLMs with reinforcement learning for interactive AI systems.
- Expertise Required:
- Model Integration: Ability to design and integrate multiple AI models into a cohesive system that addresses complex tasks.
- Cross-Disciplinary Knowledge: Understanding of multiple AI domains, enabling the creation of hybrid solutions that draw on the best features of different models.
- Application-Specific Design: Skills in tailoring hybrid models to specific use cases, such as autonomous driving, where both vision and decision-making models are crucial.
Expertise in various AI models equips businesses and developers with the tools needed to address a wide range of challenges and opportunities. From understanding the nuances of natural language processing with LLMs to deploying image recognition systems using CNNs, mastering these models enables the creation of innovative solutions that drive growth and efficiency. As AI continues to evolve, staying updated on the latest advancements across different AI models will be key to maintaining a competitive edge in the technology landscape.
Large Language Model Development Process
Developing a Large Language Model (LLM) is a complex process that involves multiple stages, from data collection to deployment. These models, like OpenAI’s GPT-4, are built on deep learning architectures that require vast amounts of data and computational power. The development process is both technically challenging and resource-intensive but yields powerful models capable of understanding and generating human-like text. Below is a detailed step-by-step guide to the LLM development process.
1. Defining Objectives and Use Cases
- Objective Setting:
- Begin by clearly defining the goals and objectives of the LLM. What specific tasks or problems will the model address? This could include tasks like text generation, summarization, translation, or customer support automation.
- Use Case Identification:
- Identify the primary use cases for the LLM. Understanding the target audience and their needs helps in guiding the model’s development, ensuring it aligns with business goals.
2. Data Collection and Preprocessing
- Data Sourcing:
- Gather a large and diverse dataset that reflects the language patterns, contexts, and nuances the model needs to learn. Sources may include books, articles, websites, and proprietary databases.
- Data Cleaning:
- Preprocess the data to remove noise, inconsistencies, and irrelevant content. This step includes tasks like tokenization, normalization, and the removal of duplicates or biased content.
- Dataset Annotation:
- In some cases, annotate the data with labels or metadata to assist in supervised learning tasks. This is especially important for fine-tuning models for specific applications.
3. Model Architecture Design
- Choosing the Architecture:
- Decide on the model architecture based on the specific requirements of the project. Transformer-based architectures are commonly used for LLMs due to their ability to process sequences of data efficiently.
- Hyperparameter Selection:
- Determine key hyperparameters, such as the number of layers, attention heads, and hidden units. These will significantly impact the model’s performance and computational requirements.
4. Training the Model
- Training Setup:
- Set up the computational environment required for training, which often involves distributed computing and specialized hardware like GPUs or TPUs.
- Model Training:
- Train the model on the collected dataset. This process involves feeding the data into the model and adjusting the weights through backpropagation and optimization algorithms (e.g., Adam optimizer).
- Handling Overfitting:
- Implement regularization techniques, such as dropout or early stopping, to prevent the model from overfitting on the training data.
- Monitoring Progress:
- Continuously monitor the training process using metrics like loss, accuracy, and perplexity. Adjust hyperparameters as necessary to improve performance.
5. Fine-Tuning the Model
- Task-Specific Fine-Tuning:
- Fine-tune the pre-trained model on a smaller, task-specific dataset to enhance its performance for specific applications, such as customer service chatbots or content generation.
- Transfer Learning:
- Leverage transfer learning by using a pre-trained LLM as the base model and fine-tuning it on a domain-specific dataset to reduce training time and resource consumption.
6. Evaluation and Testing
- Validation:
- Split the data into training, validation, and testing sets. Use the validation set to tune hyperparameters and make decisions about model architecture adjustments.
- Performance Metrics:
- Evaluate the model using metrics like BLEU (for translation), ROUGE (for summarization), or accuracy (for classification tasks). Ensure that the model meets the predefined objectives.
- Bias and Fairness Testing:
- Test the model for biases, ensuring that it does not produce unfair or discriminatory outputs. Use fairness metrics and diverse datasets for this purpose.
7. Deployment and Integration
- Model Deployment:
- Deploy the model into the production environment. This could involve setting up APIs, integrating with existing systems, or embedding the model into applications.
- Scalability Considerations:
- Ensure the deployment is scalable, with the capability to handle high volumes of requests. Techniques like model quantization or distillation can help in optimizing performance for production.
- Monitoring and Maintenance:
- Set up continuous monitoring of the model’s performance in production. This includes tracking metrics, gathering user feedback, and making necessary adjustments or retraining as required.
8. Post-Deployment Support and Iteration
- Model Updates:
- Regularly update the model with new data and retrain it to improve accuracy and adapt to evolving language patterns or user needs.
- User Feedback Integration:
- Collect and analyze user feedback to refine the model’s outputs and enhance its effectiveness in real-world applications.
- Ongoing Evaluation:
- Continuously evaluate the model’s performance, ensuring it meets the latest standards in accuracy, fairness, and ethical AI practices.
The development of a Large Language Model is a rigorous process that requires careful planning, extensive resources, and ongoing iteration. From defining objectives to deploying and maintaining the model, each step is critical to the success of the project. By following this structured development process, businesses can build robust LLMs that deliver significant value, enhancing everything from customer interactions to content creation and beyond.
Expertise implemented in LLM Development
Developing a Large Language Model (LLM) requires a multidisciplinary approach that draws on a wide range of expertise. From understanding deep learning techniques to ensuring ethical AI practices, the successful development of an LLM hinges on the seamless integration of various skill sets. Below are the key areas of expertise that are crucial to the LLM development process.
**1. Deep Learning and Neural Networks
- Architecture Design:
- Expertise in designing and optimizing neural network architectures, particularly transformer models, which form the backbone of LLMs. This involves understanding attention mechanisms, layer configurations, and the impact of hyperparameters on model performance.
- Model Training:
- Skills in training large-scale models on massive datasets using distributed computing techniques. This includes knowledge of frameworks like TensorFlow, PyTorch, and the ability to utilize specialized hardware like GPUs and TPUs.
- Optimization Techniques:
- Proficiency in implementing optimization algorithms such as Adam or SGD, and techniques like learning rate scheduling, to efficiently train models while managing computational resources.
2. Natural Language Processing (NLP)
- Text Preprocessing:
- Expertise in preparing raw text data for model training, including tokenization, stemming, lemmatization, and dealing with out-of-vocabulary words. Understanding how to create meaningful text embeddings is also crucial.
- Language Modeling:
- Knowledge of language modeling techniques that enable LLMs to predict word sequences, generate coherent text, and understand context in natural language. This includes familiarity with both autoregressive models (like GPT) and masked language models (like BERT).
- Sequence-to-Sequence Learning:
- Skills in developing and fine-tuning models for tasks like translation, summarization, and question answering, which require an understanding of how to handle input-output sequences effectively.
3. Data Engineering and Management
- Data Collection and Annotation:
- Expertise in sourcing and curating large and diverse datasets, which are essential for training LLMs. This includes managing data pipelines, ensuring data quality, and using annotation tools to label data when necessary.
- Data Augmentation:
- Knowledge of techniques for augmenting data to increase the diversity of training samples, which helps in building more robust models. This might involve synthetic data generation or techniques like paraphrasing in NLP.
- Big Data Management:
- Skills in handling and processing vast amounts of text data using big data technologies like Apache Hadoop, Spark, or cloud-based solutions. Ensuring data is processed efficiently and securely is critical.
4. Model Fine-Tuning and Transfer Learning
- Fine-Tuning Techniques:
- Proficiency in fine-tuning pre-trained models on specific datasets to adapt them to particular tasks or domains. This process involves careful adjustment of learning rates, batch sizes, and other hyperparameters.
- Transfer Learning:
- Expertise in leveraging transfer learning to reduce training time and resource requirements. This includes understanding how to use a pre-trained LLM as a base and adapting it to new tasks with minimal additional training.
5. Ethical AI and Bias Mitigation
- Bias Detection and Mitigation:
- Skills in identifying and mitigating biases in both the training data and the model’s outputs. This requires a deep understanding of the sources of bias in NLP and the implementation of fairness algorithms.
- Ethical AI Practices:
- Knowledge of ethical AI principles, including transparency, accountability, and data privacy. Implementing these principles ensures that the LLM operates within ethical boundaries and complies with regulatory standards.
- Impact Assessment:
- Expertise in assessing the societal and cultural impact of deploying LLMs, particularly in sensitive applications like healthcare, law, and finance. This includes ensuring that the model’s decisions are fair and unbiased.
6. Software Engineering and DevOps
- Model Deployment:
- Proficiency in deploying LLMs in production environments, which involves setting up APIs, managing cloud resources, and ensuring that the model can scale to handle real-world traffic. Experience with containerization (Docker, Kubernetes) and CI/CD pipelines is essential.
- Performance Optimization:
- Skills in optimizing the model for inference, including techniques like quantization, pruning, and model distillation. This ensures that the LLM can deliver fast and efficient responses in production settings.
- Monitoring and Maintenance:
- Expertise in setting up monitoring tools to track the model’s performance over time, detect drifts, and manage updates or retraining processes. Continuous integration and deployment practices ensure that the LLM remains up-to-date and effective.
7. Human-Computer Interaction (HCI)
- User Experience (UX) Design:
- Understanding of how users interact with AI-driven systems and the ability to design interfaces that facilitate seamless interactions with the LLM. This includes designing conversational interfaces, chatbots, and other NLP-driven applications.
- Interactive Learning:
- Skills in creating feedback loops where users can interact with the LLM, providing input that the model can learn from in real time, improving its responses and adaptability.
The development of a Large Language Model is a multidisciplinary endeavor that requires a confluence of expertise in deep learning, NLP, data management, ethical AI, software engineering, and user experience design. Mastery in these areas enables the creation of powerful, efficient, and responsible LLMs that can drive innovation across various industries. By integrating these diverse skills, developers can build LLMs that not only excel in their technical performance but also align with ethical standards and user needs.
LLM Solutions used across various Industries
Large Language Models (LLMs) have revolutionized numerous industries by providing powerful AI-driven solutions that enhance efficiency, automate processes, and improve decision-making. Below are some key industries where LLMs are making a significant impact, along with specific applications within each sector.
**1. Healthcare
- Medical Documentation and Summarization:
- LLMs are used to automatically generate and summarize medical documentation, reducing the administrative burden on healthcare professionals. They help in creating patient reports, clinical summaries, and discharge notes with high accuracy.
- Clinical Decision Support:
- LLMs assist healthcare providers by analyzing medical literature and patient data to offer evidence-based recommendations. They can help diagnose diseases, suggest treatment options, and predict patient outcomes.
- Patient Interaction and Support:
- Chatbots and virtual assistants powered by LLMs are deployed to handle patient inquiries, provide information about symptoms and treatments, and schedule appointments, improving patient engagement and satisfaction.
**2. Finance and Banking
- Risk Management and Compliance:
- LLMs are used to analyze vast amounts of financial data, regulatory documents, and legal texts to help institutions manage risks and ensure compliance with regulations. They can flag potential compliance issues and suggest corrective actions.
- Fraud Detection:
- By analyzing transaction patterns and customer behavior, LLMs can identify anomalies and flag potentially fraudulent activities in real-time. This improves the security of financial transactions and protects against fraud.
- Automated Customer Support:
- Financial institutions use LLMs to power chatbots that can handle a wide range of customer queries, from account management to financial advice, significantly reducing response times and operational costs.
**3. Retail and E-commerce
- Personalized Recommendations:
- LLMs analyze customer behavior, preferences, and purchase history to provide personalized product recommendations. This enhances the shopping experience and drives sales by suggesting relevant products to customers.
- Automated Content Creation:
- Retailers use LLMs to generate product descriptions, promotional content, and marketing copy at scale. This allows for faster content creation while maintaining consistency and quality.
- Customer Service Automation:
- E-commerce platforms implement LLM-powered chatbots and virtual assistants to handle customer inquiries, process orders, and resolve issues, ensuring 24/7 customer support with minimal human intervention.
**4. Legal Services
- Document Review and Contract Analysis:
- LLMs streamline the legal document review process by quickly analyzing contracts, identifying key clauses, and flagging potential risks. This reduces the time and cost associated with manual document review.
- Legal Research:
- Legal professionals use LLMs to conduct research by querying large databases of legal texts, case laws, and statutes. This speeds up the research process and ensures that lawyers have access to the most relevant and up-to-date information.
- Litigation Support:
- LLMs assist in preparing legal briefs, summarizing case documents, and predicting case outcomes based on historical data. This helps lawyers strategize and make informed decisions in litigation.
**5. Education and E-Learning
- Intelligent Tutoring Systems:
- LLMs power adaptive learning platforms that provide personalized tutoring and feedback to students. These systems adjust the difficulty of exercises based on the student’s performance and learning pace.
- Content Generation and Summarization:
- Educators use LLMs to generate educational content, create quizzes, and summarize textbooks. This saves time and allows for the rapid development of learning materials.
- Language Learning:
- LLMs are used in language learning applications to provide real-time grammar correction, vocabulary suggestions, and conversational practice, enhancing the effectiveness of language education.
**6. Media and Entertainment
- Content Creation and Scriptwriting:
- LLMs assist writers and content creators by generating ideas, drafting scripts, and suggesting dialogue. They are used in writing for films, TV shows, and online content, speeding up the creative process.
- Automated Moderation:
- Media platforms use LLMs to automatically moderate user-generated content, filtering out inappropriate language, hate speech, and spam, ensuring a safe and positive environment for users.
- Audience Engagement:
- LLMs analyze audience behavior and preferences to tailor content recommendations, improving user engagement and retention. They also help in crafting personalized messages and interactive experiences.
**7. Customer Support and CRM
- Automated Responses:
- Companies deploy LLMs in customer relationship management (CRM) systems to automate responses to common customer inquiries, resolving issues more quickly and freeing up human agents for more complex tasks.
- Sentiment Analysis:
- LLMs are used to analyze customer feedback, reviews, and social media mentions to gauge customer sentiment. This helps businesses understand customer satisfaction and identify areas for improvement.
- Chatbots and Virtual Assistants:
- LLM-powered chatbots provide round-the-clock customer support, handling tasks like order tracking, troubleshooting, and providing product information, enhancing the overall customer experience.
**8. Human Resources and Recruitment
- Resume Screening:
- LLMs streamline the recruitment process by automatically screening resumes, matching candidate qualifications with job descriptions, and shortlisting the most suitable candidates for further evaluation.
- Employee Onboarding:
- HR departments use LLMs to create personalized onboarding experiences, providing new hires with the information and resources they need to get started, as well as answering common questions.
- Employee Engagement:
- LLMs analyze employee feedback, survey responses, and performance data to assess engagement levels and identify potential issues. This helps HR teams to improve workplace satisfaction and retention.
Large Language Models have become a transformative force across various industries, driving innovation and improving operational efficiency. From healthcare to finance, retail to legal services, LLMs offer solutions that not only automate routine tasks but also enhance decision-making, customer engagement, and overall business performance. By leveraging LLMs, businesses can stay competitive and deliver better outcomes in an increasingly AI-driven world.
Key Takeaways:
- Transformative Impact: LLMs are revolutionizing industries by enhancing customer interactions, automating complex tasks, and providing valuable insights. From healthcare and finance to retail and legal services, their applications are diverse and impactful.
- Enhanced Efficiency: By automating routine processes and analyzing vast amounts of data, LLMs streamline operations, reduce costs, and improve accuracy. This leads to significant time savings and allows professionals to focus on higher-value tasks.
- Personalization and Engagement: LLMs enable highly personalized experiences by analyzing user behavior and preferences. This is particularly evident in areas like e-commerce and education, where tailored recommendations and adaptive learning improve user satisfaction and engagement.
- Ethical Considerations: As powerful as LLMs are, their deployment must be guided by ethical principles. Ensuring fairness, mitigating biases, and maintaining transparency are crucial for responsible AI development and deployment.
- Future Potential: The potential of LLMs continues to grow as technology evolves. Advances in model architecture, training techniques, and computational power will further enhance their capabilities, leading to even more innovative applications and solutions.
ConclusionLarge Language Models (LLMs) represent a groundbreaking advancement in artificial intelligence, reshaping industries and driving significant progress across various domains. Their ability to understand and generate human-like text has opened up new possibilities for automation, efficiency, and innovation.
In conclusion, Large Language Models are not just technological marvels but also powerful tools that drive efficiency, innovation, and growth across various industries. Embracing and leveraging these models can provide a competitive edge, enabling businesses to harness the full potential of AI and shape the future of their respective fields.

Large Language Models (LLM) Development: A Comprehensive Guide to Their Development in 2024 was originally published in Coinmonks on Medium, where people are continuing the conversation by highlighting and responding to this story.